AssemblyNet-AD-FTD
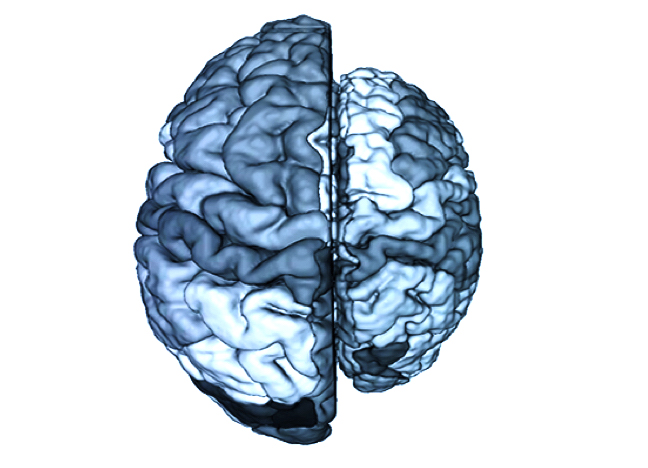
AssemblyNet-AD-FTD is a pipeline designed to automatically detect/predict dementia in a T1w brain MRI. It gets an anonymized MRI brain volume in NIFTI format and produces a pdf report containing structure grading scores.
AssemblyNet-AD-FTD produces a probability score based on deep learning models trained to discriminate Alzheimer's disease (AD) and Frontotemporal Dementia (FTD) signatures. The grading map provides a score for each structure that reflects the similarity of the subject under study to a population with AD or FTD (i.e., if the T1w MRI content presents AD-like or FTD-like patterns). In addition, AssemblyNet-AD-FTD combined deep grading based features with volume-based features to establish the final diagnosis.
All the considered structures are segmented using AssemblyNet
Be aware that AssemblyNet-AD-FTD has been designed only to discriminate AD and FTD patients from controls. Do not use it for other types of pathologies (e.g., Parkinson, Vascular dementia, ...). Moreover, the definition of AD follows ADNI and the definition of FTD follows the clinical classification proposed by NIFD. Only three sub-types of FTD are considered: behavioral variant FTD (bvFTD), semantic variant (SV) and progressive nonfluent aphasia (PNFA).

Report
Once the process is finished you will be notified by e-mail so you will be able to download a package including some image files and two (CSV and PDF) reports presenting the probability for the three considered classes (i.e., CN, AD and FTD). Moreover, the report provides a grading map indicating the most impacted structures as well as their proximity to the three considered classes.
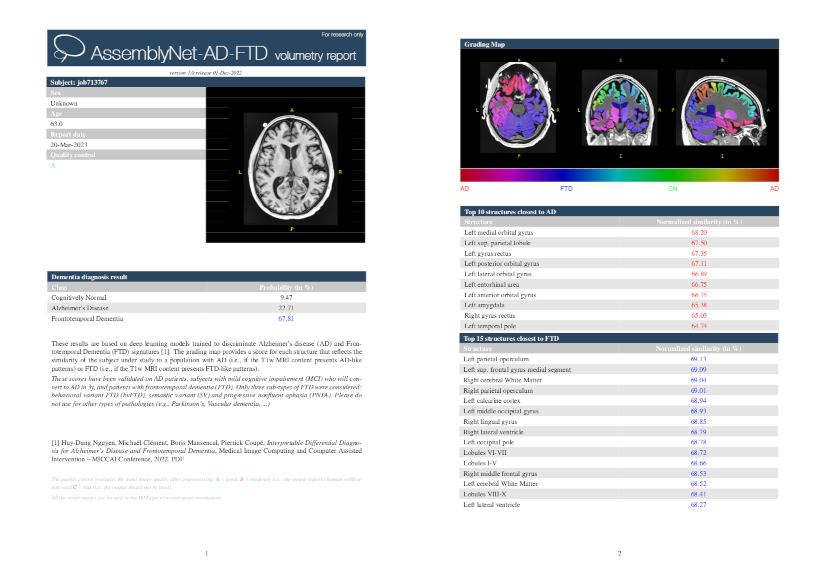
References
Nguyen, H.D., Clément, M., Planche, V., Mansencal, B. and Coupé, P., 2023. Deep grading for MRI-based differential diagnosis of Alzheimer’s disease and Frontotemporal dementia. Artificial Intelligence in Medicine, 144, p.102636. PDF
P. Coupé, B. Mansencal, M. Clément, R. Giraud, B. Denis de Senneville, V.-T Ta, V. Lepetit, J. V. Manjon. AssemblyNet: A large ensemble of CNNs for 3D Whole Brain MRI Segmentation. NeuroImage, 219, 117026, 2020. PDF
de Senneville, B.D., Manjon, J.V. and Coupé, P., 2020. RegQCNET: Deep quality control for image-to-template brain MRI affine registration. Physics in Medicine & Biology, 65(22), p.225022. PDF